The Rise of AI—and Its Unexpected Flaws
The last few years have seen an explosion in the adoption of AI tools, especially Large Language Models (LLMs) like OpenAI’s GPT-4, Google’s Gemini, and Anthropic’s Claude. These models are increasingly integrated into enterprise workflows—from customer service automation and sales enablement to compliance reporting and internal knowledge management.
According to McKinsey’s 2023 State of AI report, over 60% of companies are now using AI in some capacity, and more than a quarter are using generative AI tools regularly. However, along with their growing impact comes a significant and often misunderstood flaw: AI hallucinations. These are not merely technical glitches—they represent one of the biggest threats to trust, compliance, and operational reliability in enterprise AI systems.
AI hallucinations occur when an AI system produces factually incorrect or entirely fabricated outputs that appear convincing and well-structured. This can be as benign as a wrong date or as serious as a made-up financial policy in a customer support interaction. Enterprises that fail to recognize and mitigate this issue risk damaging their brand reputation, alienating customers, and even facing legal consequences.
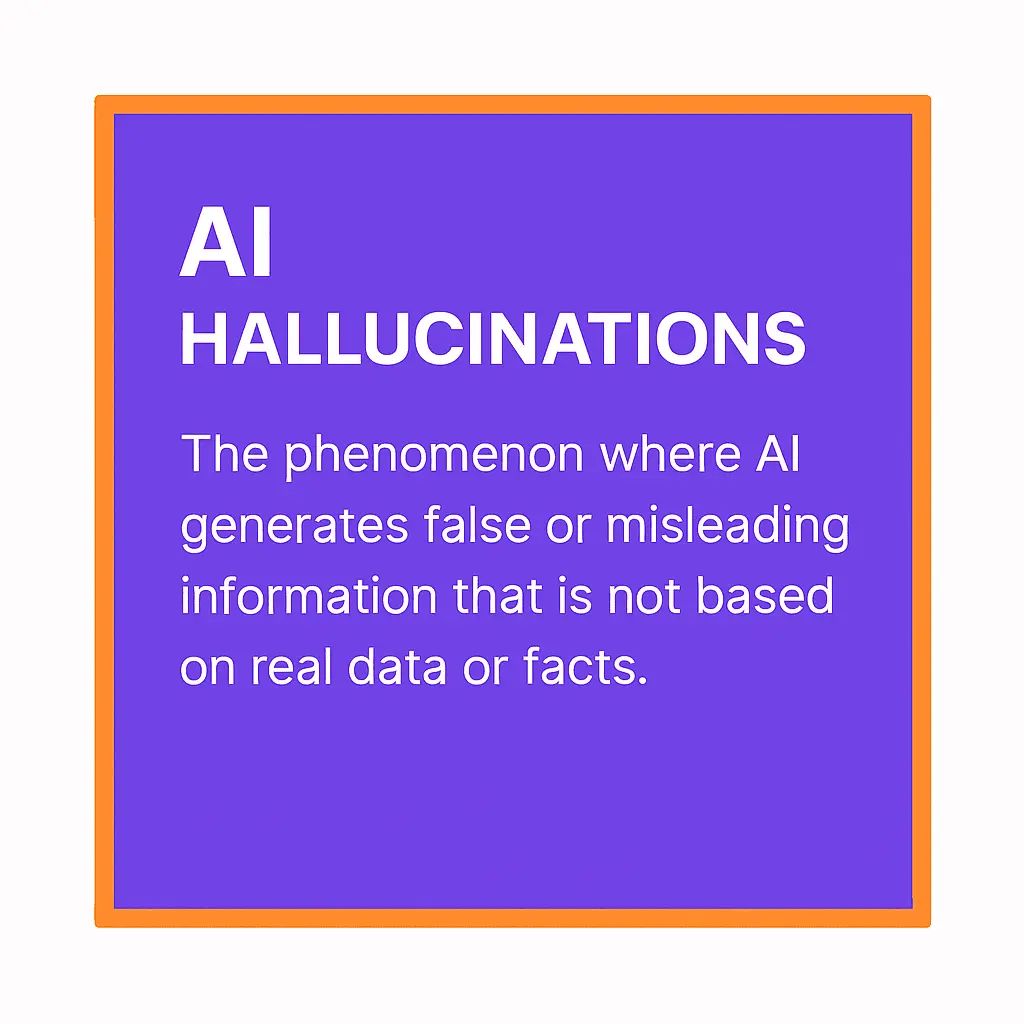
What Are AI Hallucinations?
AI hallucinations are outputs generated by language models that are not grounded in real-world facts or data. These responses often sound confident and articulate, making them deceptively persuasive and difficult to identify.
Key Characteristics:
- Fabricated URLs, statistics, or company policies
- Incorrect product descriptions or instructions
- Misattribution of sources or quotes
For example, a chatbot powered by a general-purpose LLM may confidently direct a customer to a non-existent returns page or cite a made-up Forbes article. These kinds of LLM response errors are not malicious but stem from the underlying probabilistic nature of how LLMs generate text.
Unlike human misinformation, which can be intentional or due to ignorance, hallucinations are a byproduct of prediction-based outputs without sufficient context or grounding.
Why Do AI Models Hallucinate?
Understanding the technical causes of hallucination is key to solving the problem. Here’s a breakdown of why this happens:
- Prediction-Driven Outputs: At their core, LLMs predict the next word based on prior patterns seen during training. If the training data lacked accurate information about a topic, the model may “fill in the blanks” with plausible—but false—details.
- Lack of Grounding: Most LLMs do not connect to a real-time database or external truth source during inference. They generate responses based on what they’ve learned from static training data, which can become outdated.
- Prompt Sensitivity: A small change in phrasing can result in dramatically different outputs. This makes consistency difficult and hallucinations more likely.
- Overfitting to Patterns: In some cases, LLMs learn patterns that are statistically likely but factually wrong, such as referencing a popular but incorrect internet myth.
Recent research from Stanford University (2023) found that GPT-4 hallucinates in approximately 19% of factual tasks when not grounded by external tools like retrieval systems.
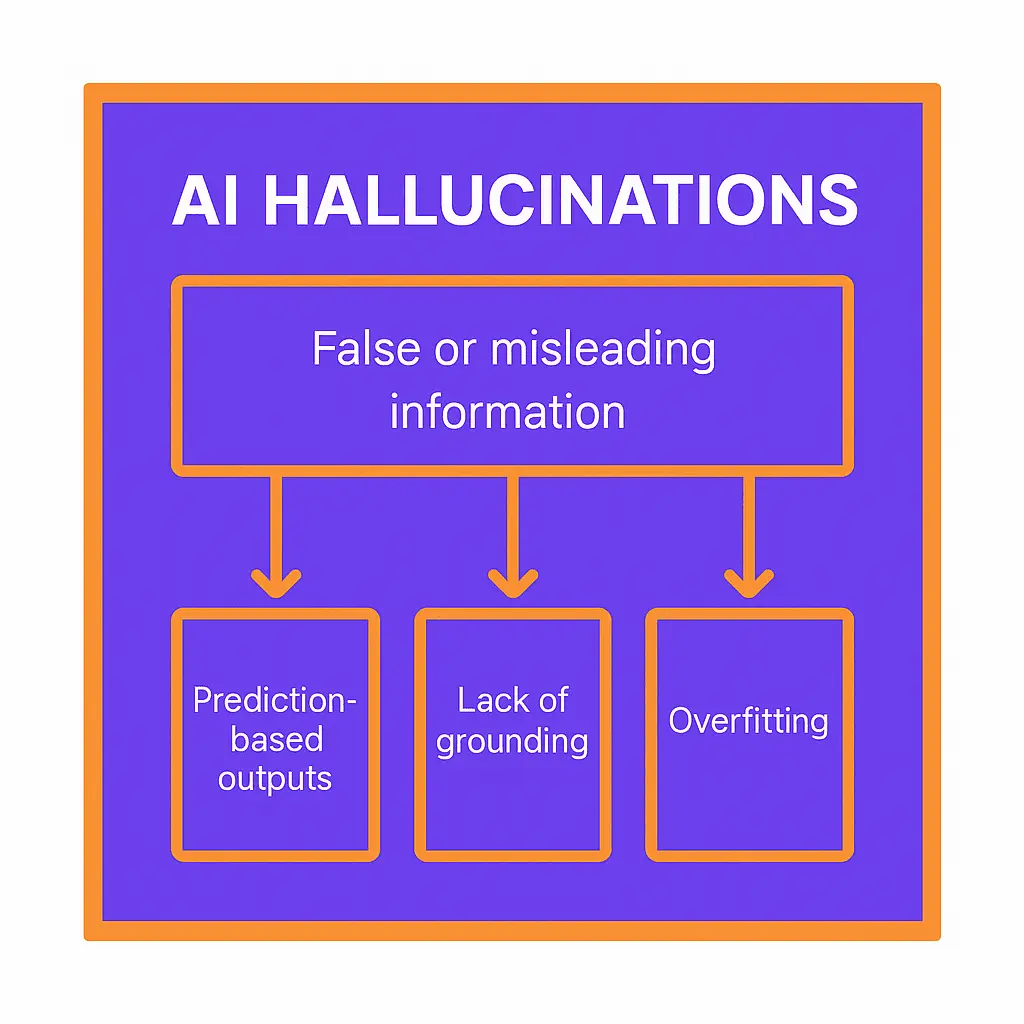
The Risks of AI Hallucinations in Enterprise Applications
The implications of hallucinations in enterprise settings are far-reaching and can directly impact business operations and customer experience:
- Loss of Customer Trust: Imagine a bank chatbot incorrectly stating that a loan is eligible for 0% interest. When the customer finds out the real policy, it leads to frustration, potential churn, or even social media backlash.
- Legal and Compliance Violations: In regulated sectors like finance, healthcare, and insurance, misinformation can lead to breaches of compliance laws, attracting fines or lawsuits. The EU AI Act and similar regulations are increasingly focusing on model transparency and accuracy.
- Operational Inefficiencies: Internally, AI tools used for knowledge retrieval or employee training may offer incorrect procedures or outdated policy references, slowing down workflows and requiring manual intervention.
Reputation Damage: In 2023, a Fortune 500 financial institution faced public scrutiny when its AI advisor recommended high-risk investments to risk-averse clients. Though unintentional, the hallucinated advice led to a major PR crisis and a temporary shutdown of the pilot.
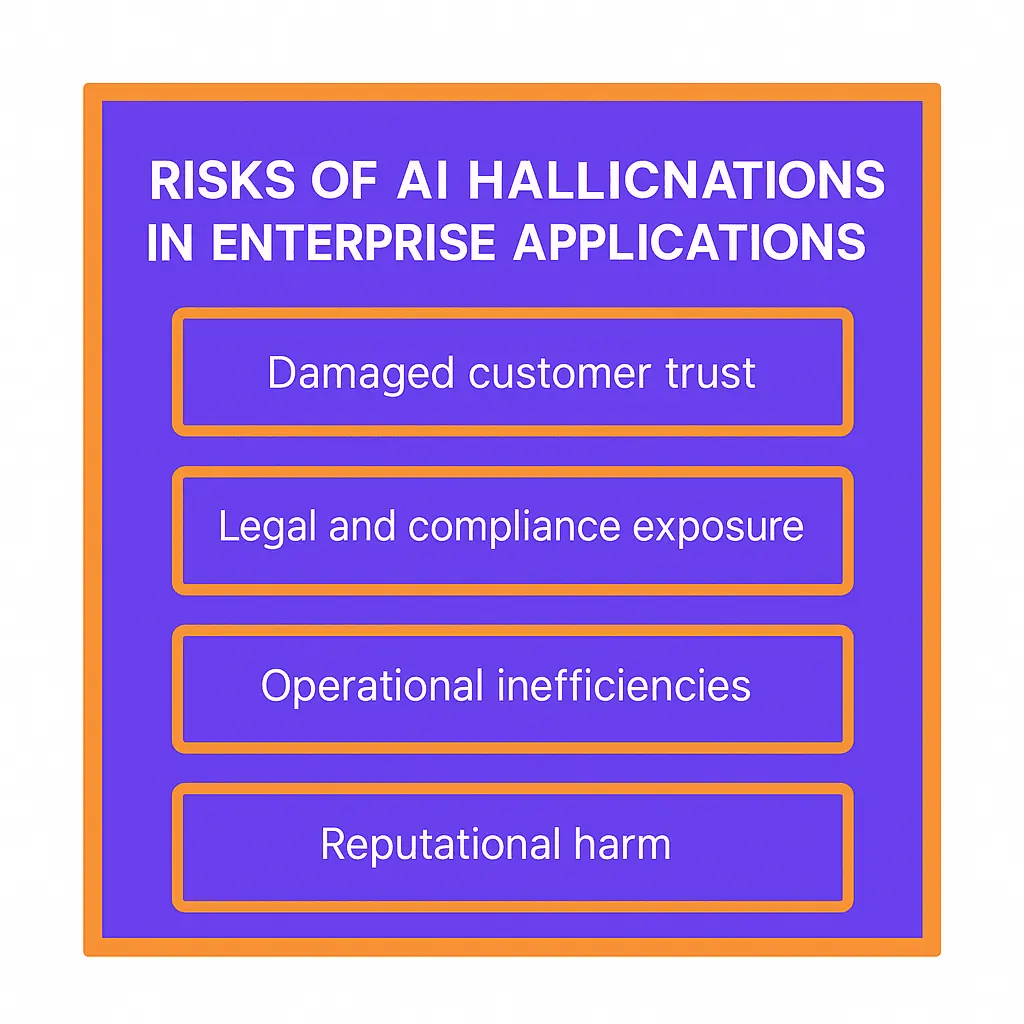
How Enterprises Can Detect and Mitigate AI Hallucinations
No AI system is infallible, but enterprises can build safeguards that minimize the risk and impact of hallucinations:
a. Human-in-the-Loop (HITL):
Deploying AI systems with human reviewers, especially for high-impact decisions, ensures critical outputs are verified. Human oversight is particularly important in sectors like healthcare and finance.
b. Fine-Tuning with Domain-Specific Data:
Customizing models with proprietary datasets reduces reliance on general internet knowledge. This boosts accuracy and aligns outputs with enterprise standards.
c. Retrieval-Augmented Generation (RAG):
RAG combines generative models with search engines or databases to pull real-time, factual data into responses. For example, OpenAI’s ChatGPT with browsing or tools like LangChain provide this functionality.
d. Confidence Scoring and Fallback Protocols:
Models can assign confidence levels to outputs. When scores fall below a threshold, systems can default to simpler FAQ responses or escalate to a human.
e. Feedback Loops and Monitoring:
Collect user feedback and log hallucination incidents. Over time, use these logs to retrain models and improve robustness.
Microsoft and IBM have implemented such multi-layered systems in their enterprise AI tools to deliver grounded, verifiable responses.
Building Trustworthy AI Systems: Best Practices for IT Leaders and Product Managers
Creating reliable AI tools goes beyond engineering. It’s about strategic design and governance:
a. Transparency and Explainability:
Choose vendors and platforms that offer explainable AI outputs. Tools like Google’s Vertex AI and Amazon Bedrock now offer interpretability dashboards.
b. Testing at Scale:
Conduct rigorous scenario-based QA using real-world prompts. Stress-test the system under ambiguous queries to identify edge-case vulnerabilities.
c. Hybrid Architectures:
Combine deterministic systems (e.g., rule-based engines) with generative AI to control high-risk interactions. Salesforce’s Einstein GPT is a good example of a hybrid architecture.
d. User Education:
Train employees and end-users to identify suspicious outputs. A well-informed user is an important layer of defense.
e. Regulatory Readiness:
Stay aligned with global AI governance standards, from the EU AI Act to the U.S. Blueprint for an AI Bill of Rights.
Conclusion: AI Is Powerful, But Not Infallible
AI is revolutionizing enterprise productivity, but it is not a truth engine. AI hallucinations are a fundamental limitation of LLMs and must be treated as a core design consideration—not an afterthought.
By investing in safeguards like RAG, fine-tuning, and hybrid workflows, enterprises can significantly reduce the risks. The goal is not to eliminate hallucinations entirely, but to make them manageable, traceable, and correctable.
If your organization is deploying AI, now is the time to consult your vendor or AI team about hallucination mitigation strategies. Don’t wait for a hallucination to become a headline. Build trust from the start.
DaveAI’s GRYD framework is purpose-built to prevent AI hallucinations by integrating structured knowledge delivery with real-time context adaptation. GRYD combines retrieval-augmented generation (RAG) with a modular, enterprise-aligned knowledge graph to ensure responses are grounded in verified business data—not just model predictions. It dynamically curates content from authenticated sources such as product catalogues, FAQs, and CRM systems, reducing the likelihood of fabricated or inconsistent answers. By orchestrating the flow of content through intelligent orchestration layers, GRYD enforces factual accuracy, relevance, and consistency—making it a robust safeguard against hallucinations in enterprise-grade AI deployments.
FAQs
No. Hallucinations are unintended and typically arise from model design, while misinformation may be spread intentionally.
No. But they can be significantly reduced using grounding techniques, monitoring, and domain-specific fine-tuning.
Start with RAG, integrate HITL validation, monitor outputs rigorously, and invest in staff training.
Absolutely not. Instead, treat hallucinations as a manageable engineering challenge. With the right strategy, AI can safely enhance enterprise performance.